I. MED-SuMo: searching, browsing and superposing 3D macromolecules structure
Macromolecules surface similarity detection with MED-SuMo at PDB scale is a technology breakthrough to discover a wealth of drug design data. MED-SuMo is a state of the art technology to search into 3D databases, find similar binding surfaces and generate 3D superpositions based on common surface chemical features and similar shape.
MED-SuMo is a software for searching, browsing and superposing 3D macromolecule structures:
- Protein kinases, serine proteases, phosphatases, aspartic proteases …
- Non trivial superpositions of structures from different families or different superfamilies (Fig.1)

II. Site mining with MED-SuMo: searching, browsing and superposing 3D macromolecules structures:
Superposing protein active sites of a given superfamily (protein phosphatase, protein kinases, serine proteases …) helps to deciphering the contributions to affinity and selectivity of the protein’s surface chemical features. This binding site characterization illuminates Conformational diversity and Functionnal diversity
The method applies to all protein superfamilies highly represented in the PDB like the protein kinases, serine proteases, phosphodiesterases, HSP90, beta secretase, protein phosphatase…
Searching the PDB with a PDE4 binding site:
In this application, MED-SuMo is used to explore the difference between two phosphodiesterases PDE4 and PDE5. Their catalytic domain are highly conserved, especially for PDE4 and PDE5 which is not surprising since they address very close ligand: cAMP and cGMP. The MED-SuMo Graphical User interface helps to compare one PDE structure to the whole PDB. to view superpositions and to analyse the signatures of the binding sites (Fig.2).
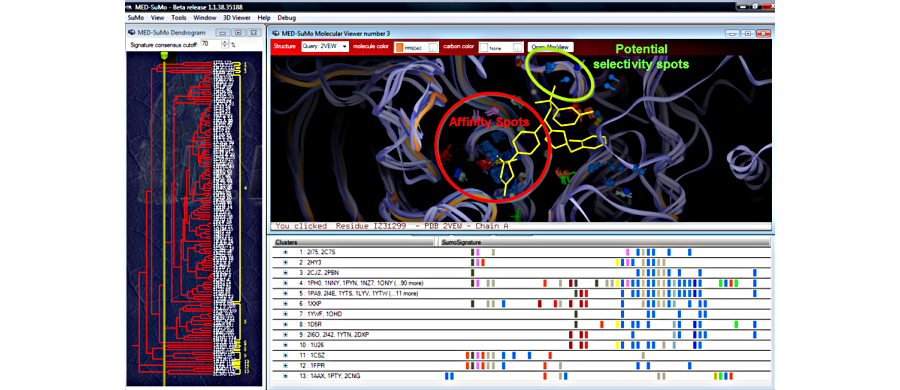
Analysis of the results: higlighting the importance of rotamers of GLN 443 residue:
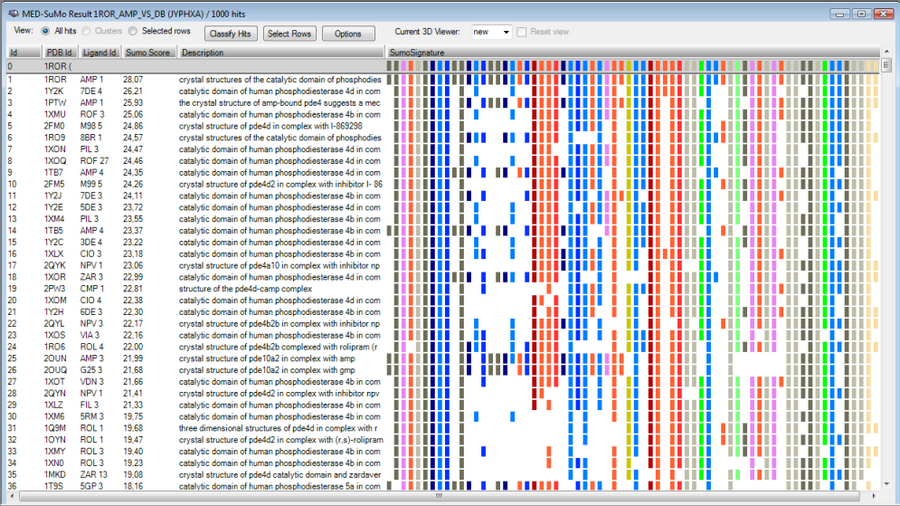
In this application, we’ve compared the PDE4-cAMP complexe (PDB code 1ROR) to the whole PDB. The first hit, which is a PDE5, is 1T9S and is ranked 36th just after many PDE4 and 2 PDE10 (click on the image on the left to enlarge). The score of 1T9S, is high (18.2) and corresponds to 65% of the maximum score achievable (exact match to the query). This indicates that PDE4 and PDE5 share most of their active site. The main difference is found at the Tyr403, which is a different amino acid in the PDE5 and is not directly involved in the interaction with the ligand.
Another interesting point is the absence, for PDE5, of the Surface Chemical Features which represents the amide function of the Gln443 of PDE4 (This SCF is green). It is known that in the PDE4-cAMP interaction the nucleotide is recognized by a bidentate H-bond motif involving Gln443, a residue conserved across all PDE families. The rotation of this critical amide side chain is fixed by a network of additional H bonds.
The recognition of a guanine (as in cGMP) instead of adenine (in the cAMP), as in cGMP-specific PDE5, goes with the rotation which reverses the amide side chain (inside the yellow ellipse, figure on the left) allowing to meet the inverted H-bond of the lactam of guanine. If we look at the 3D superimposition of 1ROR and 1T9S we can see that MED-SuMo is able to detect this structural difference between PDE4 and PDE5 (on the left). There isn’t an amide SuMo object in the PDE5 signature because SuMo detects the different position of the Gln side chain. MED-SuMo characterizes in term of chemical function in 3D the active site of the PDE.
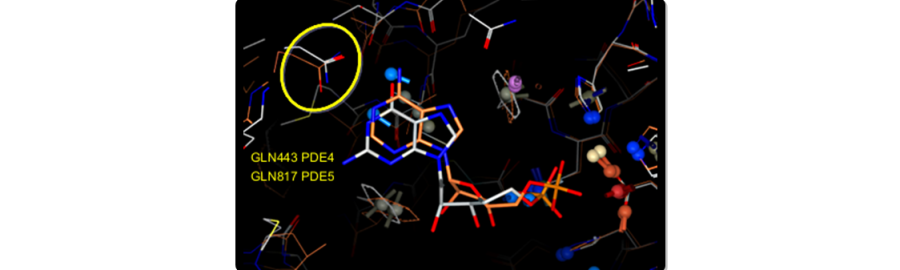
To know if this difference between PDE4 and PDE5 is always conserved with different ligands, a PDE5 complexed with vardenafil is used as a second query (PDB code: 1XP0) towards the whole PDB. The results show that the hits at the top of the list are all PDE5 followed by PDE4 (click on the image on the left to enlarge). It can be seen that the SuMo object which represents the amide function of the Gln817 in the query is not present in the signatures of the PDE4s. It can also be seen in the result table that the PDE5 structure 2H44 misses the GLN817 amide object as well, the visualisation of the superposition indicates that they are rotated by about 90 degrees.
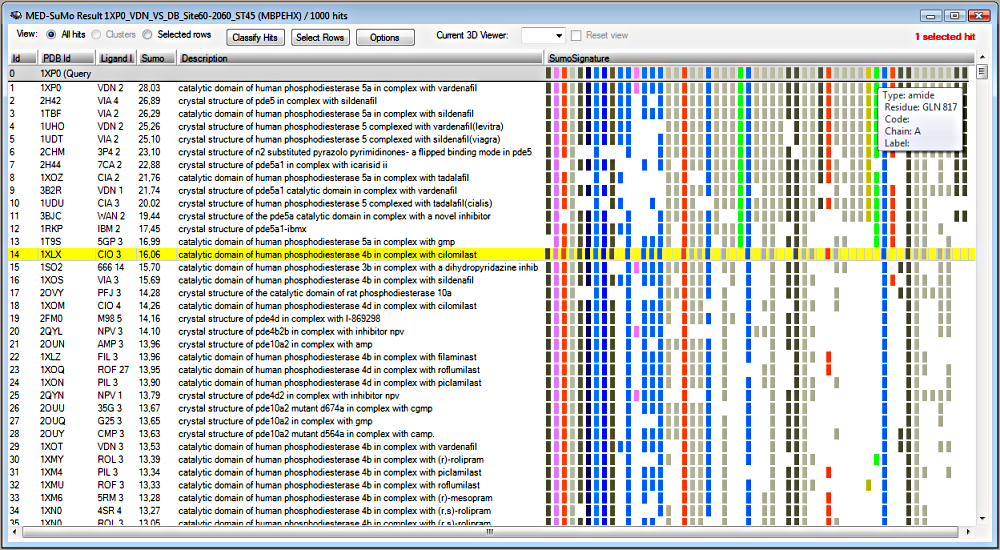
III. Drug repositioning: similar sites are likely to bind the same ligand
Repurposing tested small molecules drugs for new indications or new mechanism of action is an appealing strategy. MED-SuMo can be used in cases where a drug is co-cristalized with its target. If a similar binding site can be found in the Protein Data Bank [1] (or any macromolecule structure database), it is likely that this drug would also bind to this similar target. This target hopping case is on one hand probably rare but, on another hand, could be a strong rational evidence to highlight a possible off-target and eventually a possible undesired side effect. During lead discovery for a new target, finding cross-reactivity to a target for which there are already leads, enables the fast discovery of new leads via target-hopping. With the potential of short-circuiting the lead discovery process on a genomic scale, target hopping is an important chemogenomic application of structural informatics.
MED-SuMo is used in Site vs Binding Site database to find in the PDB the most similar sites to B-RAF/sorafenib:
This case study is about an example of repurposing sorafenib from B-RAF to others protein kinases. The B-RAF-sorafenib complex 3D structure is available in the PDB (code 1UWH [2]) and is used as the input of MED-SuMo to query the PDB binding site database. The database is redundant in terms of unique site or pocket but very interestingly for our application, it is exhaustive in term of kinase conformations and bound ligands.
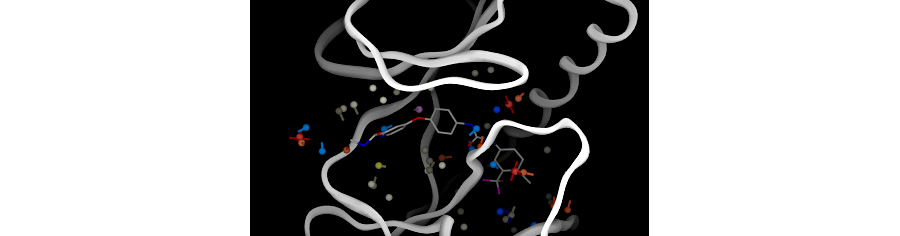
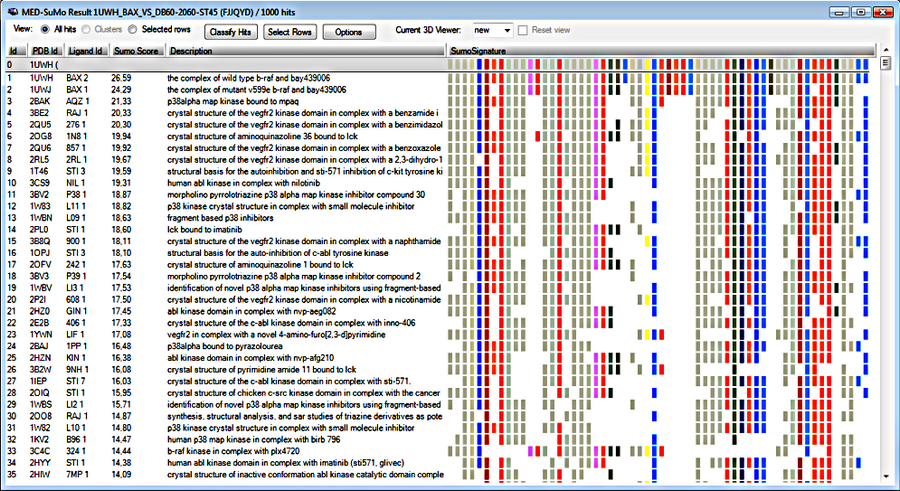
A simple measure of similarity between the site hit and the query site is obtained by normalizing the score of the hit to the maximum possible score (site query towards itself)) : Relative MED-SuMo score (%) = Hit score / Query score * 100
In this case study, we show that target hopping is likely to occur above a relative MED-SuMo score of 60%: the first hit which is not a DFG-out kinase is a DFG-in protein kinase: 3C4C ranked as the 33th hit. The relative score is 54% which makes sense as the DFG-in and the DFG-out binding mode share the hinge and the gatekeeper region which is about 50% of the pocket.
Validation of the prediction of sorafenib repurposing:
B-RAF and C-Kit have a common ligand, sorafenib (BAY439006), a drug on the market since 2006, which is an oral inhibitor of C-RAF, wild-type B-RAF, mutant V599E BRAF, vascular endothelial growth factor receptor VEGFR2, VEGFR3, FLT-3, platelet-derived growth factor receptor, p38, and C-KIT among other kinases [3]. More recently, sorafenib was shown to inhibit several protein kinases [4]: B-RAF: 540 nM, P38α: 370 nM, VEGFR2: 59 nM, LCK: 2700 nM, ABL1: 680 nM, C-KIT: 31 nM, Tie2: 2100 nM and others. The 3D binding site similarity between B-RAF and C-KIT had been highlighted previously by Debe et al. [5]. The authors pointed out the fact that the cross-reactivity of B-RAF and C-KIT can be rationalized by the 3D similarity of the binding site and not by sequence alignments because 1/6 of kinases are more similar to B-RAF than C-KIT. In the MED-SuMo results (Tab1), C-KIT is one of the best ranked (PDB code 1T46 [6]): 9th hit and 4th protein kinase after B-RAF, P38α, VEGFR2, LCK and ABL1. Interestingly, MED-SuMo points out, as top ranked hits, targets which are experimentally validated. This application is case where the concept that similar targets (sequence and conformation) can bind the same ligand with a similar binding mode.

Tie2 is found among the hits with at a relative MED-SuMo score of 44% (below the 60% cutoff). Even if the conformation of 2OSC is DFG-out, like the query, the differences in the binding site (ATP pocket) are detected and a score significantly below 60% is found. This hit could be seen as a false negative if hte results are analysed with the cutoff value without further molecular modeling.
Though no experimental data are available to our knowledge, sorafenib is predicted to inhibit chicken SRC. This is likely to occur on a structural basis because the 2OIQ structure is DFG-out and contains imatinib which has the same binding mode as sorafenib. Interestingly, sorafenib is reported to be a low affinity binder to human SRC [6]. Further work would be needed to check if the boarderline relative score of 60% found with chicken SRC is a prediction of a lower experimental affinity.
Conclusion:
Starting from a B-RAF/sorafenib complex available in the PDB, MED-SuMo found, as top ranked, 5 protein kinases which are known experimentally to bind sorafenib: VEGFR2, P38α, ABL, C-KIT and LCK. MED-SuMo provides true positives and no false positives. False negatives could occur for two reasons: (1) lack of a similar structure in the PDB (in this case study, a DFG-out structure is needed) (2) sorafenib binds to others protein kinase with another binding mode. MED-SuMo is best suited to detect off-targets, though not all off targets can be detected. Interestingly, the detected off-targets with a high relative score higher than 60% are very likely to be real off-targets in vitro.
The complex of sorafenib with those 5 targets can be easily exported for further molecular modeling/scoring.
References:
[1] H.M. Berman, J. Westbrook, Z. Feng, G. Gilliland, T.N. Bhat, H. Weissig, I.N. Shindyalov, P.E. Bourne: “The Protein Data Bank” (2000) Nucleic Acids Research, 28 pp. 235-242.
[2] Wan, P.T., Garnett, M.J., Roe, S.M., Lee, S., Niculescu-Duvaz, D., Good, V.M., Jones, C.M., Marshall, C.J., Springer, C.J., Barford, D., Marais, R. “Mechanism of activation of the RAF-ERK signaling pathway by oncogenic mutations of B-RAF” 2004 Cell 116: 855-867
[3] Ahmad T, Eisen T. “Kinase inhibition with BAY 43-9006 in renal cell carcinoma” (2004) Clin Cancer Res. 10(18 Pt 2):6388S-92S.
[4] Karaman MW, Herrgard S, Treiber DK, Gallant P, Atteridge CE, Campbell BT, Chan KW, Ciceri P, Davis MI, Edeen PT, Faraoni R, Floyd M, Hunt JP, Lockhart DJ, Milanov ZV, Morrison MJ, Pallares G, Patel HK, Pritchard S, Wodicka LM, Zarrinkar PP. A quantitative analysis of kinase inhibitor selectivity. Nat Biotechnol. 2008 Jan;26(1):127-32.
[5] Debe D.A., Hambly K. P., Danzer J.F. “Structural informatics: chemogenomics in silico” in Chemogenomics, knowledge-based approaches to Drug Discovery (2006) edited by Edgar Jacoby (Novartis Institutes for Biomedical Research, Switzerland)
[6] Mol, C.D., Dougan, D.R., Schneider, T.R., Skene, R.J., Kraus, M.L., Scheibe, D.N., Snell, G.P., Zou, H., Sang, B.C., Wilson, K.P. “Structural basis for the autoinhibition and STI-571 inhibition of c-Kit tyrosine kinase.” (2004) J.Biol.Chem. 279: 31655-31663
IV. Fragment Based approach
MEDIT is offering a new computational drug design protocol combining local similarity of protein surfaces and a fragment-based approach [1-6]. It is based on MED-SuMo and brings together their respective advantages in an attractive way. The protocol is intended for fragment library design, lead discovery and lead optimization. Lead Discovery and Lead optimization applications are performed with MED-Hybridise.
Fragment-based drug discovery has emerged in the last decade and is in contrast to conventional high throughput screening (HTS) where fully built, “drug-sized” chemical compounds are screened for activity. Small chemical structures or fragments (100-250 Da) that intrinsically have weaker binding affinity (100 mM to 10 nM range) are screened to probe the complete binding site, and then to identify larger molecules based on one or multiple binding fragments.
Obtaining experimental structural information on fragments or ligands complexed to a target protein is a key element and also a major limitation to the number and types of target that are amenable to fragment-based drug discovery. Consequently, computational methods play a key role in deriving structural information for designing compounds that fit a particular site on a given protein
MEDP-Fragmentor and MED-Sumo to get pool of aligned fragments in an input binding site:
MED-SuMo enables populating binding sites by searching and retrieving MED-portions chemical moieties from the MED-SuMo fragment database. This database of MED-Portions , where a MED-Portion is a new structural object encoding protein-fragment binding sites, is generated with MED-Fragmentor. a collected pool of MED-Portion chemical moities is shown below in the case of a DFG-out protein kinase pocket:
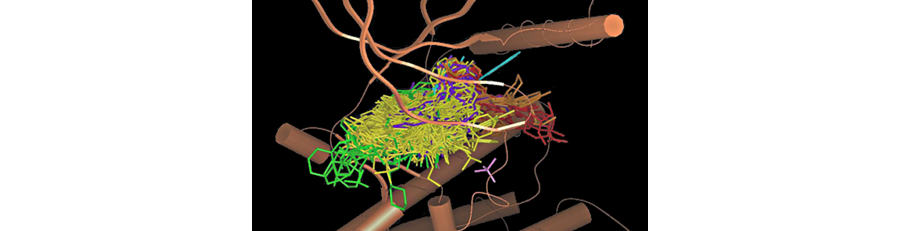
Binding sites superimposition with MED-SuMo leads to ligands alignments:
In our fragment-based approach protocol, we do not hybridise ligands like is was done in a pioneering work published in 2004 [7] (Figure 7.) because the retrieved ligands are found to be much more likely to have strong bumps (steric clashes) with the query protein than the smaller MED-Portion chemical moieties [Moriaud2009]. After a bump count between the retrieved ligands and the query protein it can be concluded that this sort of starting material is not suitable for further study, since too many bumps are present.

Our strategy: MED-Portion chemical moieties hopping instead of ligand hopping from one target to another:
- Similar protein surfaces are likely to bind the same MED-Portion chemical moiety
- MED-Portion chemical moieties have smaller interaction surfaces than ligands and therefore target hopping is more likely to occur
- Many interfamily hits occur: GPCR binding sites can be successfully populated [Moriaud2009]
This work is based on two publications:
Computational fragment-based drug design to explore the hydrophobic sub-pocket of the mitotic kinesin Eg5 allosteric binding site. Oguievetskaia K, Martin-Chanas L, Vorotyntsev A, Doppelt-Azeroual O, Brotel X, Adcock SA, de Brevern AG, Delfaud F, Moriaud F. J Comput Aided Mol Des. 2009 Jun 17. PMID: 19533373
Computational fragment-based approach at PDB scale by protein local similarity. Moriaud F, Doppelt-Azeroual O, Martin L, Oguievetskaia K, Koch K, Vorotyntsev A, Adcock SA, Delfaud F. J Chem Inf Model. 2009 Feb;49(2):280-94. PMID: 19434830
PDF 2007 poster “A computational protocol to Fragment-Based Drug Design at PDB scale”
V. Site Classification at PDB scale:
MED-SuMo has an interesting and original approach to detect structural and functional similarities between protein binding sites [1-3]. We decided to use its ability to classify datasets of structures. This new method is called MED-SuMo_Multi Approach (MED-SMA renamed in MEDP-SiteClassifier) [4-6]. It enables the comparison of all the binding sites of a dataset using a pairwise comparison system. To then build a similarity matrix which is classified with Markov Clustering algorithm.
To begin, a list of proteins is selected. Then, two strategies can be adopted to create the MED-SuMo database: (i) the database contains all binding sites of the selected proteins, (i.e. binding sites where the co-crystallized ligands obey to certain prefixed rules (maximum (or minimum) number of atoms, number of residues if it is a small peptide…)); (ii) the database contains only specified binding sites (i.e. only ATP binding sites).
Once the database is created, the pairwise comparison is launched using MED-SuMo comparison procedure. These comparisons outlines the similar SCF between pairs of binding sites. Groups of SCFs between binding sites are gathered; they formed patches. Patches associated to the same binding sites are analyzed: if two patches share enough SCFs (threshold named covering factor), they are merged in a multipatch. Multipatches represent the true meaningful common regions of binding sites. They ensured two properties: (i) enough SCFs are in common, i.e., binding sites are really similar and (ii) they output subpockets similarity. To compute the similarity Matrix, the MED-SuMo score between matching multipatches is calculated. At the end, MCL interprets the matrix and classifies the protein binding site dataset into clusters of sub-sites. A 2D plot of the clusters can be visualized using Biolayout [7-8].
Application to protein binding sites which are clearly structurally and functionally different: serine proteases, kinases and lectins:
We show that the binding sites are correctly classified. Classification of an unrelated protein binding sites subset: serine proteases, kinases and lectins. MED-SuMo/MEDP-SiteClassifier classifies perfectly each family into 3 separate clusters in a short computing time (26 seconds on a single CPU).
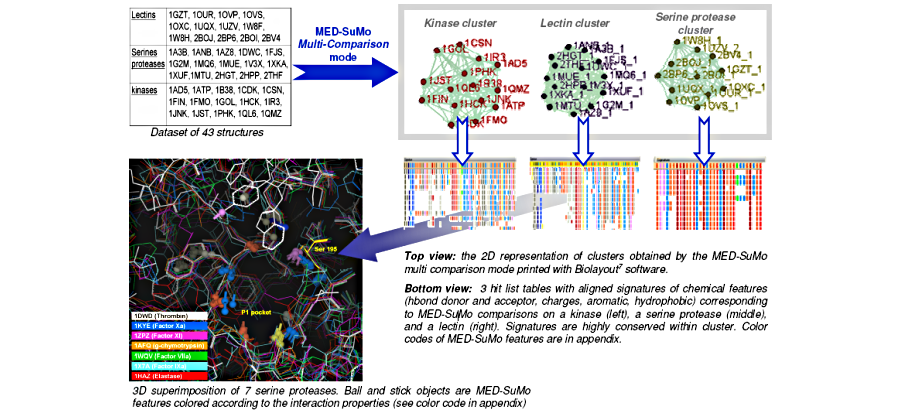
Application to protein binding sites families which are related as they bind the same ligand ATP: HSP90, Topoisomerase, HSP70, mutL, HSP70, Actin, Kinesin:
200 structures are classified in a short computing time (10 minutes on a single CPU). The families are grouped into clusters which are interconnected in some cases.
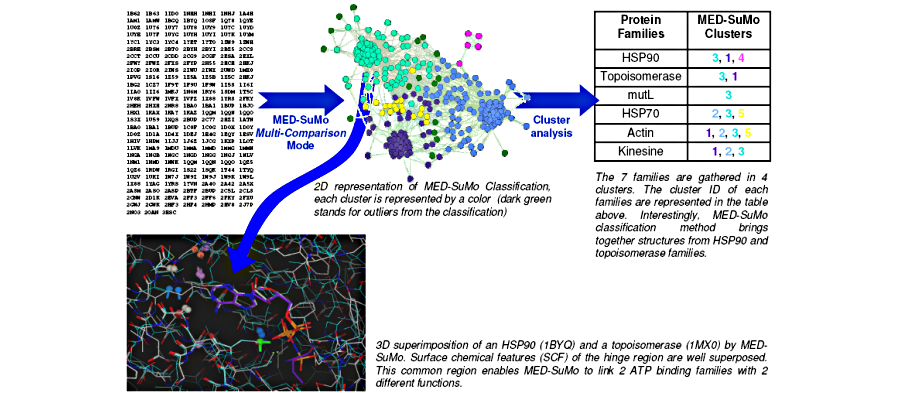
Conclusion:
The 2 case studies presented here highlight MED-SuMo/MEDP-SiteClassifier efficiency to classify structural subsets. MED-SuMo can not only separate families (first case study) that are not related but it also indicates functional links between related ones (second case study). In the protein data bank, topoisomerase and HSP90 are shown to have the same binding mode with two co-cristallized structures resolved with the same ligand RADICOL. Here we outline the link between these two families using MEDP-SiteClassifier. In addition to the short computing time (10’ on 1CPU to treat 200 structures), this finding gives perspective to our classification method.
In 2008/2009, we are applying this fast and accurate approach to classify all the binding sites of the PDB In the POPS (Peta Operation Per Second) collaborative project.
MED-PSiteClassifier PDF brochure
References:
[1] Jambon M., Imberty A., Deleage G., Geourjon C. (2003) A new bioinformatic approach to detect common 3D sites in protein structures, Proteins, 52:137-134.
[2] Jambon M., Andrieu O., Combet C., Deleage G., Delfaud F., Geourjon C. (2005) The SuMo server: 3D search for protein functional sites, Bioinformatic, 21:3929-3930.
[3] Doppelt O., Moriaud F., Bornot A., de Brevern A.G. (2007) Functionnal annotation for protein structures, Bioinformation, 1:357-359
[4] Doppelt O. et al. “Classification of binding sites with MED-SuMo: application to the purinome” to be published
[5] Olivia Doppelt, Julien Castillan, Olivier Andrieu, Alexandre G. de Brevern and Fabrice Moriaud, A new functional classification method based on local protein surface comparison using MED-SuMo software, GGMM, 2007, Grenoble, France.
[7] Enright AJ, Ouzounis CA. “BioLayout–an automatic graph layout algorithm for similarity visualization.” Bioinformatics. 2001 Sep;17(9):853-4.
[6] Olivia Doppelt, Julien Castillan, François Delfaud, Alexandre G. de Brevern and Fabrice Moriaud, Structural Classification of diverse binding sites using 3D Surface chemical features
[8] Goldovsky L, Cases I, Enright AJ, Ouzounis CA. “BioLayout(Java): versatile network visualisation of structural and functional relationships.” Appl Bioinformatics. 2005;4(1):71-4.
VI. Epitope scaffold search
How to search proteins having a given 3D secondary structure in the Protein Data Bank ? Let’s say that you have the structure of an antigen, it could be a few residues or as large as a protein-protein interaction surface. You will want to identify proteins than have the same 3D secondary structure on its surface. The search is based on finding a protein match between query and hit with similar positions of alpha carbon and orientation of the sidechains.
To focus on the most relevant results, compute and check if the proximate chains in the query (chains bound to the epitope) are likely to bind without too many bumps to the hit proteins.
So you will identify from the PDB, proteins likely to have similar interaction as the one in the query. Please note that the kind of sidechain is not checked in this tutorial, so you can identify hits with similar residues or not.
Ofek et al. searched the PDB for mimotopes of HIV1 gp41 epitope.They have reported 5 protein structures as parents of epitope scaffolds of the gp41 epitope. They designed epitope scaffolds from those parents, like ES2 from 1KU2 PDB file (Thermus aquaticus RNA Polymerase Sigma Subunit Fragment). They have shown the interest of searching mimotopes of GP41. In this tutorial, we show how MED-SuMo can retrieve the same computational results and also many others proteins from the PDB which are likely to be candidate for Protein Vaccine (with some protein engeneering on the sidechains.
The Query is built from 1TJI PDB file (released in 2004) and is the gp41 epitope (ELLELDKWASL) bound to the antibody 2F5. Then the whole PDB is mined for this epitope. This video is describing the search of epitope scaffold and the analysis of the results:
This work is based on the following publication : Ofek G, Guenaga FJ, Schief WR, Skinner J, Baker D, Wyatt R, Kwong PD. Elicitation of structure-specific antibodies by epitope scaffolds. Proc Natl Acad Sci U S A. 2010 Oct 19;107(42):17880-7. PMID: 20876137